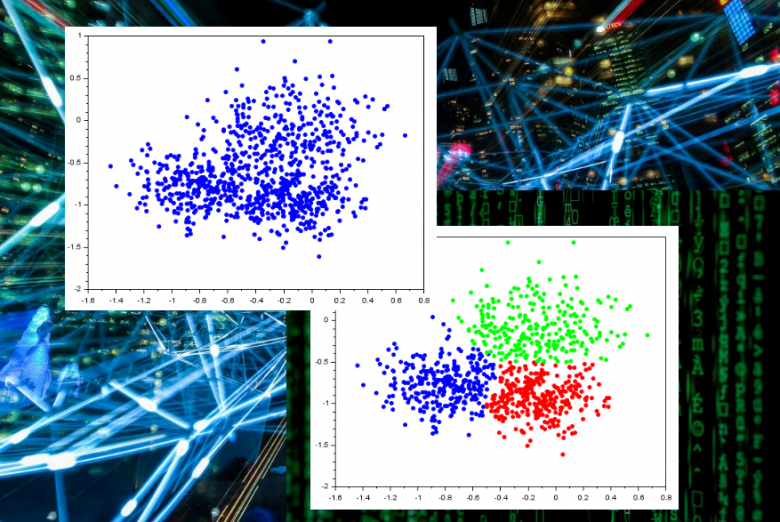
Peutz frequently carries out large monitoring projects. Over a longer period of time for instance sound and/or vibrations are being measured, resulting in a large amount of data. Due to the size and variation in the data caused by various sources, the analysis of these data is complex and time consuming. With new machine learning techniques more information can be obtained from the data than is possible with conventional statistical or manual analysis. With a clustering algorithm the data is divided into natural clusters and for each separate cluster the statistics can be obtained.
Algorithm determines noise level freight traffic
During sound monitoring all spectral data are being saved in a database. An algorithm can search in this spectral information for sound characteristics that often occur. In this way disturbances can be grouped in clusters and through investigating samples it is easily determined which sound source is dominant in each cluster. This for instance can be trucks, airplanes, cooling machines, wind turbines, etc.. Because the algorithm clusters all data of each specific sound source, better insight is gained in the relative contribution of this sound source to the overall occurring sound pressure levels. It can be determined which part of the time sound originating from a specific sound source occurs in the day, evening and night period, so that for each period the relative contribution can be determined. In the figure below, a visualisation is given of the relative contribution of a specific sound source. This concerns a case in which the contribution of freight traffic in the occurring sound pressure levels near a projected hotel is determined through the use of an algorithm. From this it can be concluded that in the night period almost no freight traffic occurs, which means that less drastic measures regarding soundproofing of facades are needed.
Through the use of machine learning algorithms for the analysis of monitoring data more information can be obtained from the data. This results in more detailed insights which can be used for more well-founded decisions regarding infrastructural, industrial, building technical, or environmental issues. This can for instance mean that financial savings are possible because certain measures are not needed.
If you have any questions? Please contact our advisors Robbert-Jan Dikken or Simon de Koning.