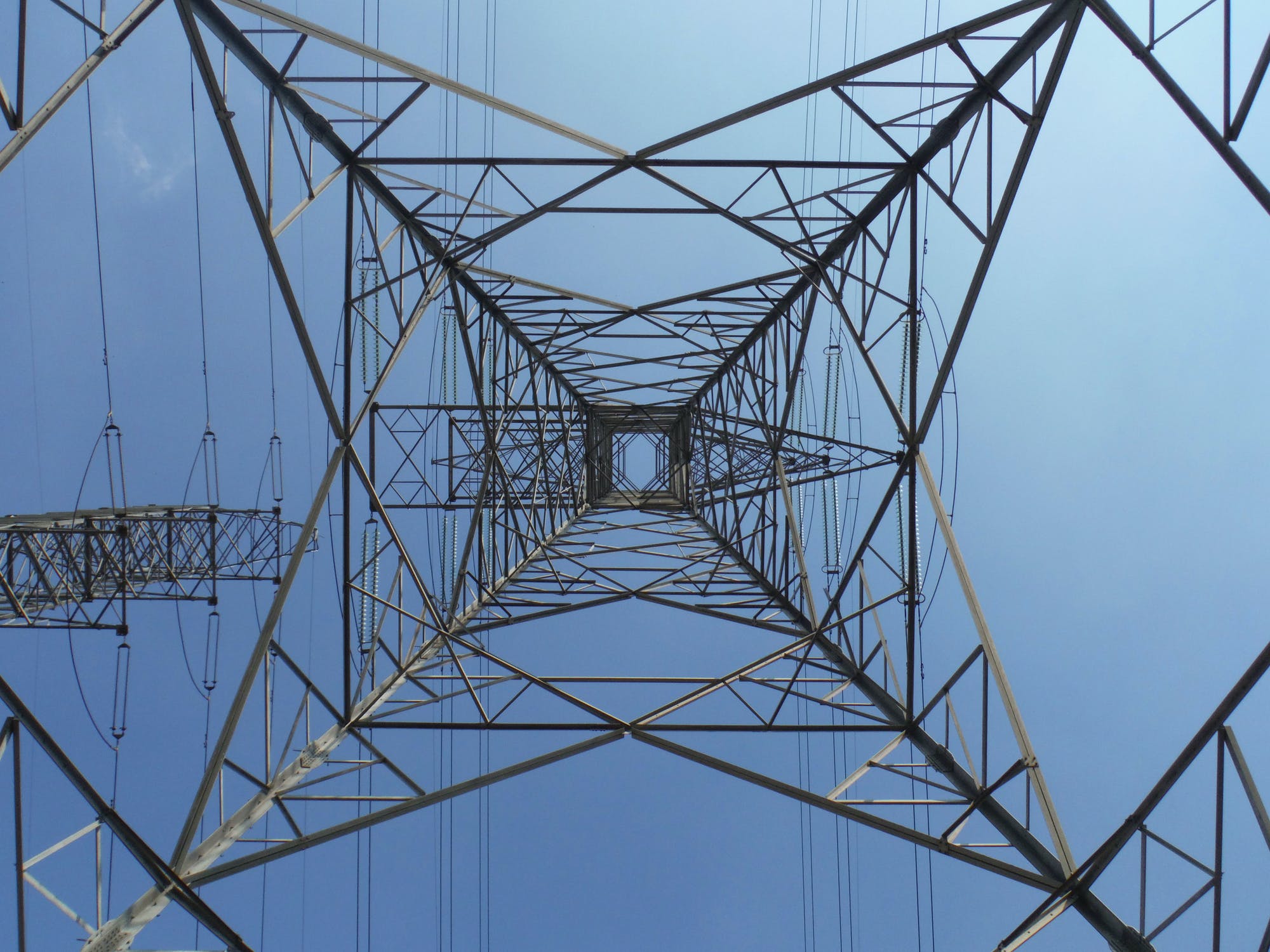
The imminent consequences of climate change for society have initiated an energy transition. The focus for energy production slowly shifts from fossil fuels to sustainable energy sources like wind and solar energy. Because of this a fundamental change in the supply of energy arises. Sustainable energy sources have a strongly fluctuating nature, as for instance solar or wind. This means that demand and supply of energy can be out of balance. This can lead to instability in power grids, which can be measured by the grid frequency that goes up when there is more supply than demand and goes down when the situation is reversed. This is obviously not desirable. Therefore it is important to be able to signal under which circumstances instability arises, so that can be acted upon this [1].
predictability of the imbalance
A lot of academic research is being done in predicting the occurrence of instability in power grids [2,3]. Such studies provide data sets that become more and more publicly available. Therefore research into the development of data-driven models which can be used to balance demand and supply of energy by predicting under which circumstances instabilities arise can be performed. Peutz constantly carries out studies how physics-based and data-driven models can contribute to societal issues. Recently we have performed a study based on a publicly available data set into the predictability of the imbalance in a power grid with one energy supplier and three consumers. This concerns a data set that results from an academic test case and consists of information on the supplied and consumed power, reaction times and price dependencies. A correlation analysis shows no evident correlation between the separate parameters and the stability, as depicted in the correlation matrix.
Stability prediction
The mentioned parameters have a non-trivial relation with the stability of the grid. Considering the success with which machine learning (ML), and specifically artificial neural networks, are applied the last few years for non-trivial problems, the applicability for this classification problem (stable/unstable) is being studied. This is being studied with two different methods: 1) stability prediction with a single ML model (a neural network); 2) stability prediction with a committee machine (a collective of neural networks). For the first method a neural network is trained directly on a part of the data set and tested independently on another part of the data set. For the second method multiple neural networks are being trained on a part of the data set, and subsequently the collective of neural networks is being used to produce an expectation value for the stability on an independent test set. In the two tables the percentage of correct and incorrect predictions for both classes (stable/unstable) is given for both the single model and the committee machine. When these two methods are being compared with each other it appears that the committee machine performs significantly better than a single neural network. A single neural network has a reasonably good accuracy, but the committee machine improves the accuracy with 4 to 5 percent with respect to the single model.
With these kind of collective data-driven models it can be predicted accurately under which circumstances instability will arise in a power grid, so that better strategies for energy production, consumption and storage can be determined.
Wisdom of the crowd
From previous studies carried out by Peutz it appears that the application of a committee machine, i.e. a collective of models, often performs better than a single model for complex problems. This is analogous to the concept "wisdom of the crowd", for which a crowd can give a better judgment with respect to a certain problem than individuals. Errors are being averaged out, so that the accuracy improves. The method of committee machines is broadly applicable and gives for classification and regression problems next to an expectation value also a measure of deviation leading to insight into the reliability of the models. This method is also for instance applied for the prediction of the sound insertion loss of attenuators in air ducts, the prediction of the electric energy usage of households and the prediction of wind nuisance in urban environments.
If you want more information about the application of data-driven models for energy related problems or what the application of machine learning in general can mean for you please do not hesitate to contact us.
Literature
[1] M. Cruciani, Electric Networks and Energy Transition in Europe, Notes de l'Ifri, September 2015.
[2] B. Schäfer et al., Taming instabilities in power grid networks by decentralized control, The European Physical Journal Special Topics 225.3, 2016, 569-582.
[3] V. Arzamasov et al., Towards Concise Models of Grid Stability, Communications, Control, and Computing Technologies for Smart Grids (SmartGridComm), 2018 IEEE International Conference on. IEEE, 2018 (Section V-A). Contact: Robbert-Jan Dikken r.dikken [at] peutz.nl