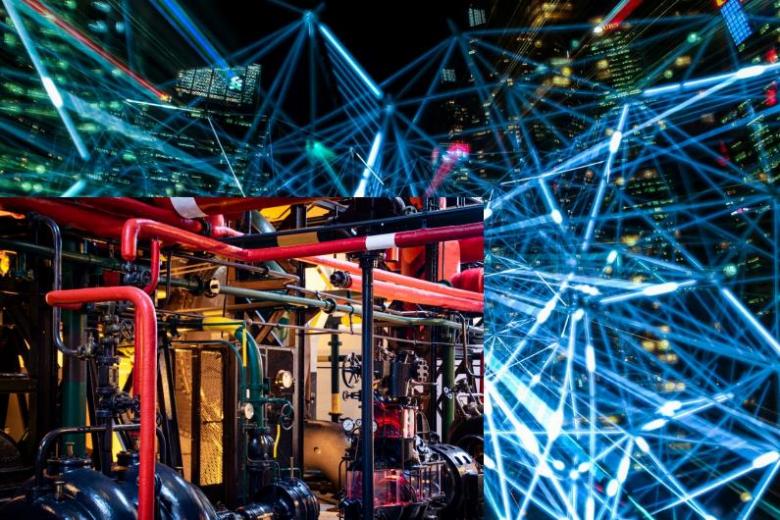
Installations have a pivotal role in the vital processes within our society. Therefore it is of great importance that installations function reliably. Nowadays many aspects of installations are being monitored using sensors. These monitoring data provide interesting opportunities in the area of maintenance in for instance the industrial, infrastructural and buildings sector, but is certainly not yet fully utilized in all cases. Although there is a shift noticeable in the direction of condition based and predictive maintenance, the conventional method of maintenance, based on statistics (average time to failure etc.), is still prevailing. However, this has a very rigid character. The availability of data in combination with advanced machine learning algorithms makes it possible to develop dynamic maintenance plans, with an anticipating character. This can lead to significant reduction in maintenance cost and down time.
So data can be used with the help of machine learning models to predict whether maintenance of an installation is needed. Obviously this means that the data has to be reliable. However, this is certainly not always the case. This can have several causes, like malfunctioning of sensors or incorrect labelling in registrations. Because machine learning models are trained with data, this also has an effect on the reliability of the predictive model. Therefore it is desired to remove incorrect data from the dataset before training a model. However, for complex problems this is far from trivial. Because many aspects are involved it is often not possible to detect and remove large outliers with classical statistical methods.
In order to develop a method to train reliable models with which predictive maintenance plans can be constructed, we studied a dataset representing the functioning of water pumps. This dataset consists of a large number of variables (like water quality, quantity, etc.) in order to predict eventually whether a water pump is functioning as it should, is functioning but needing of repair, or is not functioning at all. In this study it quickly became clear that a part of the dataset was unreliable. We developed a self-consistent method in which a predictive model is trained, which is iteratively used to remove incorrect data and to restore wrongly removed data. This process is checked through a correlation analysis. Through this process the dataset is slowly being cleaned and a model is trained with which reliable predictions of required maintenance can be made. This research is summarized in an article and published on the scientific repository HAL archives.
Publicatie HAL archives: read or donwload the publication here(pdf)
For more information or questions, please contact our advisor Robbert-Jan Dikken.